How Machine Learning Improves Business Analytics
How Machine Learning Improves Business Analytics
How Machine Learning Improves Business Analytics
Overview of Machine Learning
In today's fast-paced business environment, the advent of technology has brought about a transformative change, particularly through the realm of machine learning (ML). At its core, machine learning is a subset of artificial intelligence that employs algorithms to analyze data, identify patterns, and make decisions with minimal human intervention. What sets ML apart from traditional data analysis methods? The key lies in its ability to learn and improve over time. As more data is collected, machine learning models become increasingly accurate, adapting to new trends and insights. For instance, consider a retail company utilizing machine learning to analyze customer purchasing behavior. By sifting through vast datasets, the model can predict what products might be popular next season, allowing businesses to manage inventory precisely. Here are a few important aspects of ML in business:
- Automation: It automates repetitive tasks, allowing professionals to focus on more strategic decisions.
- Scalability: ML can handle large volumes of data without sacrificing performance, making it suited for evolving market demands.
- Predictive Power: It forecasts future trends based on historical data, giving businesses a competitive edge.
Machine learning is not merely a buzzword; it's a powerful tool that is redefining how businesses operate and make decisions.
Importance of Business Analytics
Now, let’s delve into why business analytics, especially when powered by machine learning, is crucial for organizations today. Business analytics refers to the skills, technologies, practices, and applications for continuous iterative investigation of past business performance. This practice helps organizations gain deeper insights into their operations. Imagine you're a business analyst in charge of a marketing campaign. You have access to historical data about your customers' preferences. By utilizing advanced analytics techniques, you can foresee customer behavior and tailor your marketing strategy accordingly. The significance of business analytics can be summarized as follows:
- Data-Driven Decisions: It helps organizations make informed decisions based on actual data rather than guesswork.
- Efficiency Improvement: By analyzing operational data, businesses can streamline processes and reduce costs.
- Enhanced Customer Understanding: Analytics enables companies to gain insights into customer behaviors, allowing for personalized marketing efforts that increase engagement.
In the ever-evolving landscape, the combination of machine learning and business analytics allows organizations to stay one step ahead. As a professional in this field, embracing these technologies will position you favorably in your career, enabling you to drive innovation and contribute significant value to your organization. It’s not just about staying relevant; it’s about being a leader in this data-driven world.
The Role of Machine Learning in Data Collection and Processing
Automation of Data Collection
As we dive deeper into the intersection of machine learning and data analytics, one key area that deserves attention is the automation of data collection. In a world awash with information, the ability to gather relevant, high-quality data efficiently is critical for businesses looking to thrive. Machine learning algorithms can significantly reduce the time and effort required for data collection, enabling organizations to focus on more strategic tasks. Let’s look at a scenario. Imagine running a company that relies on customer feedback from various sources—social media, online reviews, surveys, and direct customer interactions. Manually sifting through these countless data points can be not only time-consuming but also prone to errors. Machine learning can automate this process, employing techniques like web scraping and natural language processing to gather and analyze customer insights seamlessly. Here are some specific ways machine learning supports automated data collection:
- Web Scraping: ML algorithms can extract data from websites, searching and gathering relevant information about products, services, or customer sentiments.
- Form Processing: Optical character recognition (OCR) can be used to extract information from scanned documents and images, allowing organizations to digitize and analyze data efficiently.
- Sensor Data Integration: In the age of IoT (Internet of Things), machine learning models can integrate data from various sensors, monitoring parameters in real time and automatically updating databases.
With these automated systems, the goal is not just to collect data, but to do so in a way that fuels decision-making and drives organizational success.
Efficient Data Processing Techniques
Once data is collected, the next crucial step is processing that data efficiently. Traditional data processing methods can often be slow and labor-intensive, a fact that can hinder timely decision-making. Machine learning brings a new level of efficiency and effectiveness to this stage of the analytics pipeline. By leveraging algorithms designed to handle and transform large datasets, businesses can streamline their data processing capabilities. Here are some efficient processing techniques facilitated by machine learning:
- Data Cleaning: Machine learning automates the identification and correction of errors, inconsistencies, or missing values in datasets. This step is crucial for ensuring that the data is accurate and reliable, ultimately leading to better insights.
- Feature Engineering: Machine learning models can perform feature extraction or selection, determining which variables should be included in predictions to enhance the accuracy of analytical models.
- Real-Time Processing: With the ability to process data streams as they come in, ML algorithms can provide organizations with instantaneous insights. This capability is exceptionally advantageous in industries such as finance and e-commerce, where real-time data interpretation can result in immediate decision-making.
For example, consider a retailer using machine learning to analyze customer purchasing patterns as sales data comes in. This can allow the company to react to trends swiftly, potentially adjusting inventory and marketing strategies based on current customer behavior. Incorporating these efficient data processing techniques not only saves valuable time for analysts but also helps organizations turn raw data into actionable insights faster, empowering them to stay competitive in today's dynamic marketplace. As machine learning continues to evolve, its ability to optimize data collection and processing will undeniably shape the future of business analytics.

Enhancing Data Analysis with Machine Learning Algorithms
Predictive Analytics
As we explore how machine learning enhances data analysis, one of the most impactful applications is predictive analytics. This process leverages historical data to forecast future outcomes, enabling organizations to prepare and strategize effectively. Consider the financial industry, where machine learning algorithms can analyze vast amounts of historical market data. By identifying patterns and trends, these models can provide insights into potential market fluctuations. This capability empowers investment firms to optimize strategies and mitigate risks. Here are some key benefits of using predictive analytics in your business:
- Informed Decision-Making: Predictive analytics helps businesses anticipate challenges and opportunities, enabling leaders to make data-driven decisions instead of relying solely on intuition.
- Optimized Resource Allocation: By forecasting future scenarios, organizations can allocate resources like budgets and staff more efficiently, thus reducing waste and maximizing output.
- Proactive Strategy Development: With predictive insights, organizations can formulate proactive strategies to adapt to emerging trends, fostering a culture of agility and innovation.
In retail, for example, predictive analytics can help businesses manage inventory more effectively. Analyzing consumer purchasing patterns allows companies to anticipate customer demand, reducing excess stock and enhancing customer satisfaction.
Clustering and Segmentation
Another critical area enhanced by machine learning algorithms is clustering and segmentation. This technique involves grouping similar data points together to uncover hidden patterns and insights, which can prove invaluable for targeted marketing and customer engagement. Imagine being a marketing manager at a large e-commerce firm. By employing clustering algorithms, you can segment customers into distinct groups based on behaviors, preferences, and demographics. This level of analysis allows for more personalized marketing strategies. Here’s how clustering benefits your analysis:
- Enhanced Marketing Campaigns: With segmented data, businesses can tailor their marketing messages to specific customer groups, improving engagement and conversion rates.
- Improved Customer Understanding: Clustering reveals underlying patterns in customer behavior, providing businesses with a deeper understanding of their audience and helping in product development.
- Resource Efficiency: By identifying the most promising customer segments, companies can allocate marketing resources more effectively, focusing on high-potential groups.
For instance, Netflix uses clustering to analyze viewer preferences and suggests tailored content recommendations based on similar viewing habits. This not only enhances user experience but also increases customer retention. In summary, the integration of machine learning algorithms, particularly in predictive analytics and clustering, empowers organizations to make informed decisions, improve customer satisfaction, and optimize operational efficiency. By leveraging these advanced techniques, businesses can gain a competitive edge, driving both growth and innovation in today's data-driven landscape. The journey ahead is exciting, and those embracing these technologies will undoubtedly lead the way in their industries.
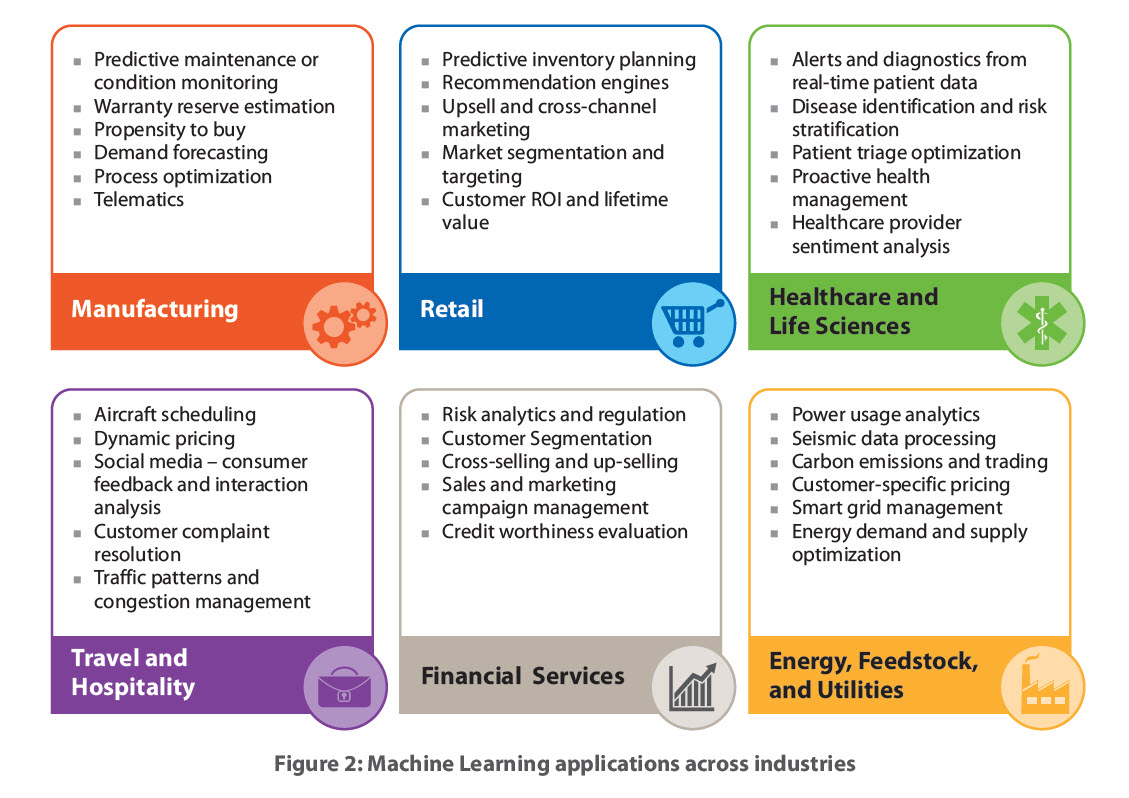
Improving Decision-Making through Machine Learning
Real-time Insights
One of the foremost advantages of machine learning in modern business is its capability to provide real-time insights. Imagine running a large retail operation where thousands of transactions occur every minute. The ability to monitor and analyze this data as it comes in can be a significant game-changer. For instance, consider a scenario where a sudden spike in online sales is detected. Machine learning algorithms can analyze patterns and identify possible reasons for this surge—be it a flash sale, social media buzz, or a trending product. Armed with these insights, decision-makers can quickly adapt their strategies. Here’s how real-time analytics empowers businesses:
- Instantaneous Decision-Making: With machine learning, leaders can make informed decisions based on current data rather than delayed reports. This immediacy is crucial in fast-paced environments like finance and e-commerce.
- Operational Efficiency: Real-time insights allow businesses to swiftly optimize operations. For example, if inventory levels are deemed insufficient during a sale, automatic alerts can trigger restock processes, through optimized supplier coordination.
- Proactive Risk Management: Machine learning models can continuously monitor for anomalies or deviations from the norm. This helps in identifying potential risks, such as fraudulent transactions, before they escalate.
On a personal note, I once witnessed a marketing team adjust their campaign ad spend based solely on real-time performance metrics delivered by machine learning models. This led to a dramatic increase in ROI, highlighting just how powerful and essential real-time insights can be in today’s data-centric business landscape.
Personalized Recommendations
The other indispensable aspect of improving decision-making through machine learning lies in its ability to deliver personalized recommendations. Customers today expect a tailored experience, and businesses that leverage machine learning to meet that expectation are at a competitive advantage. Machine learning algorithms analyze user behavior, preferences, and historical data to create personalized recommendations. For example, think of how Netflix suggests shows based on your viewing history. By applying similar logic, businesses can enhance customer engagement and loyalty. Here’s why personalized recommendations are crucial:
- Enhanced Customer Experience: Personalized messages foster a deeper connection between businesses and their customers. When customers receive recommendations that truly resonate with their interests, they are more likely to engage and make a purchase.
- Improved Conversion Rates: By targeting specific segments of your audience with tailored offers, businesses can significantly increase conversion rates. According to studies, personalized marketing can boost conversions by as much as 15-20%.
- Customer Retention: When customers feel understood and valued through customized interactions, they are less likely to churn. Retaining existing customers is often more cost-effective than acquiring new ones, making personalized service imperative for long-term growth.
From my experience in a customer-facing role, I’ve seen how targeted email campaigns driven by machine learning insights not only improved open rates but also led to higher sales volumes. This approach empowered the sales team to direct their efforts toward customers most likely to respond positively. In summary, machine learning offers invaluable tools for improving decision-making in business. By providing real-time insights and tailored recommendations, organizations can enhance operational efficiency, engage customers more profoundly, and maintain a competitive edge. As machine learning technology continues to evolve, it's clear that employing these strategies will become increasingly essential for businesses aiming for sustainable growth and success.
Machine Learning Applications in Business Analytics
Customer Behavior Analysis
In today's competitive marketplace, understanding customer behavior is paramount for businesses looking to thrive. Machine learning plays a pivotal role in unraveling the complexities of consumer actions and preferences, enabling personalized experiences that keep customers engaged. Imagine walking into a bookstore where recommendations are not just random; they align perfectly with your previous purchases and interests. This personalized experience is a product of sophisticated customer behavior analysis powered by machine learning. By leveraging historical data from various sources—such as purchase history, browsing patterns, and social media interactions—businesses can glean actionable insights into their customers' preferences. Here are some key benefits of using machine learning for customer behavior analysis:
- Enhanced Segmentation: Machine learning algorithms can segment customers based on their behaviors, identifying distinct groups with similar preferences. This enables businesses to tailor marketing campaigns specifically for each segment, increasing the chances of conversion.
- Predictive Recommendations: By analyzing customer behavior, machine learning can predict future actions. For instance, e-commerce platforms can suggest products that a customer is likely to buy next based on their shopping history. This not only drives sales but also improves customer satisfaction.
- Sentiment Analysis: Using natural language processing (NLP), machine learning can assess customer feedback and social media interactions. By gauging public sentiment towards products or services, businesses can proactively adjust their strategies to meet customer expectations.
In my experience working in marketing, we implemented machine learning models to parse through customer reviews. The insights gleaned allowed us to adjust our product features and messaging, directly enhancing our customer satisfaction scores. Such targeted adjustments can also help foster brand loyalty.
Supply Chain Optimization
Another critical application of machine learning in business analytics is supply chain optimization. This domain is complex and multifaceted, with numerous variables that can impact efficiency and costs. Machine learning provides the analytical muscle needed to navigate these challenges effectively. Consider a global retail company that needs to manage its inventory across multiple locations. Accurately predicting product demand is essential to ensure they have the right stock at the right time. By analyzing historical sales data, seasonal trends, and external factors like market conditions, machine learning algorithms can forecast future demand with impressive accuracy. The benefits of applying machine learning to supply chain optimization include:
- Improved Forecasting: Machine learning models leverage vast datasets to identify patterns in demand, allowing companies to anticipate inventory needs accurately. This capability helps prevent stockouts and reduce excess inventory, ultimately saving money.
- Logistics Optimization: Machine learning algorithms can analyze logistics data to optimize transportation routes, ensuring timely deliveries while minimizing costs. Efficient logistics can significantly enhance customer satisfaction, as timely delivery is a critical aspect of the shopping experience.
- Risk Management: By continuously monitoring supply chain variables, machine learning can detect anomalies or disruptions in the supply chain. Early detection allows businesses to mitigate risks and adapt to unforeseen changes, ensuring smoother operations.
I recall collaborating with a manufacturing client who integrated machine learning into their supply chain management. They observed a notable reduction in operational costs and improved delivery precision, resulting in happier customers and a healthier bottom line. In conclusion, the applications of machine learning in business analytics offer tremendous potential for enhancing customer insights and optimizing supply chains. By harnessing the power of data-driven insights, organizations can make strategic decisions that fuel growth, improve efficiency, and boost customer satisfaction. As machine learning continues to evolve, its role in shaping business strategies will become increasingly significant, paving the way for smarter, more agile organizations.
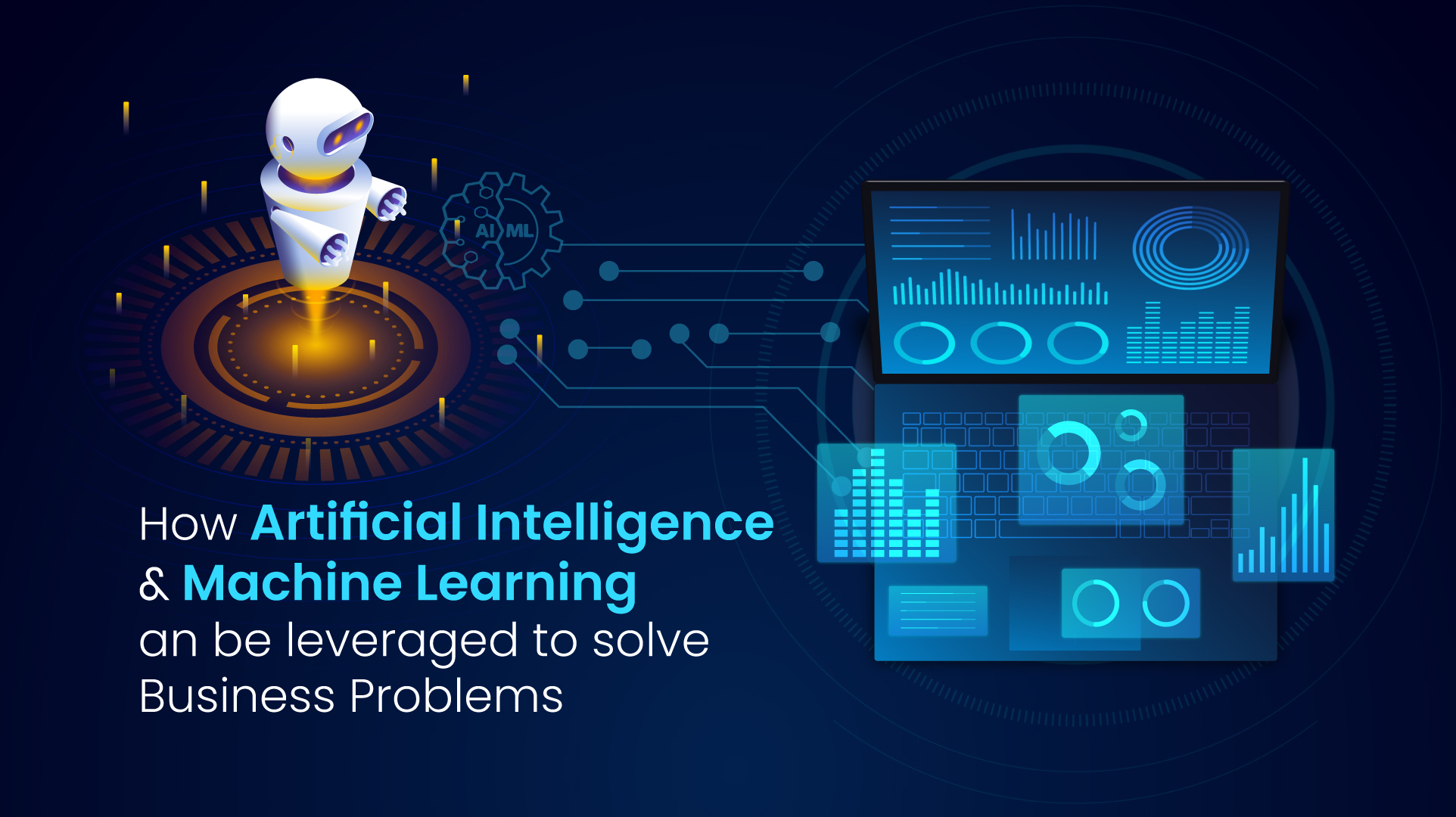
Challenges and Limitations of Implementing Machine Learning in Business Analytics
Data Privacy Concerns
As machine learning continues to reshape the landscape of business analytics, it inevitably raises important data privacy concerns. Organizations are increasingly collecting vast amounts of data to train their models, and with this comes the responsibility to handle that data ethically and securely. Imagine you're a customer of an online service that collects and analyzes your interactions to improve recommendations. While this may enhance your user experience, how much do you really know about how your data is being used? This uncertainty can lead to distrust if companies fail to communicate their data policies clearly. Here are some critical aspects that businesses need to consider regarding data privacy:
- Regulatory Compliance: With the advent of regulations like the GDPR in Europe and CCPA in California, businesses face strict requirements on how they collect, manage, and store customer data. Non-compliance can lead to hefty fines and damage to reputation.
- Data Security: Protecting sensitive information from breaches is paramount. Machine learning models can inadvertently expose vulnerabilities if not properly secured. Organizations must implement stringent data protection measures.
- Consumer Trust: Maintaining transparency with customers about how their data is utilized fosters trust. Providing users with insights into their data usage, and options to opt-in or opt-out, can enhance customer loyalty.
I’ve experienced instances where clients were hesitant to embrace machine learning solutions due to their concerns about data privacy violations. By fostering open discussions and establishing robust data governance frameworks, we were able to alleviate their fears and build trust. Navigating these privacy concerns is crucial for any organization venturing into the realm of machine learning.
Integration with Existing Systems
Another significant challenge businesses face when implementing machine learning is integrating these new technologies with existing systems. In many cases, companies already have established legacy systems that may not be compatible with advanced machine learning algorithms. This integration process can be both time-consuming and costly. Consider the following factors related to integration challenges:
- Legacy Systems: Companies often operate on outdated software that is not designed to work with modern machine learning platforms. Upgrading or replacing these systems can require significant investments of time and resources.
- Data Silos: Data often resides in separate departments or platforms, creating silos that hinder the holistic analysis required for effective machine learning. Consolidating data from various sources into a unified format can pose additional challenges.
- Technical Expertise: Successfully integrating machine learning solutions requires skilled personnel who can bridge the gap between traditional data analysis practices and modern AI technologies. The shortage of qualified professionals in this field can slow down progress.
In my own experience working on integrating a machine learning model into a client's operations, we encountered significant resistance due to their reliance on older systems. It became clear that to fully leverage the potential of machine learning, we needed to develop a clear roadmap for system upgrades and staff training. In conclusion, while the potential of machine learning in business analytics is immense, organizations must navigate the challenges of data privacy and system integration with care. By prioritizing data governance and strategically planning system transitions, businesses can harness the power of machine learning safely and effectively, ultimately unlocking valuable insights that drive growth and innovation.
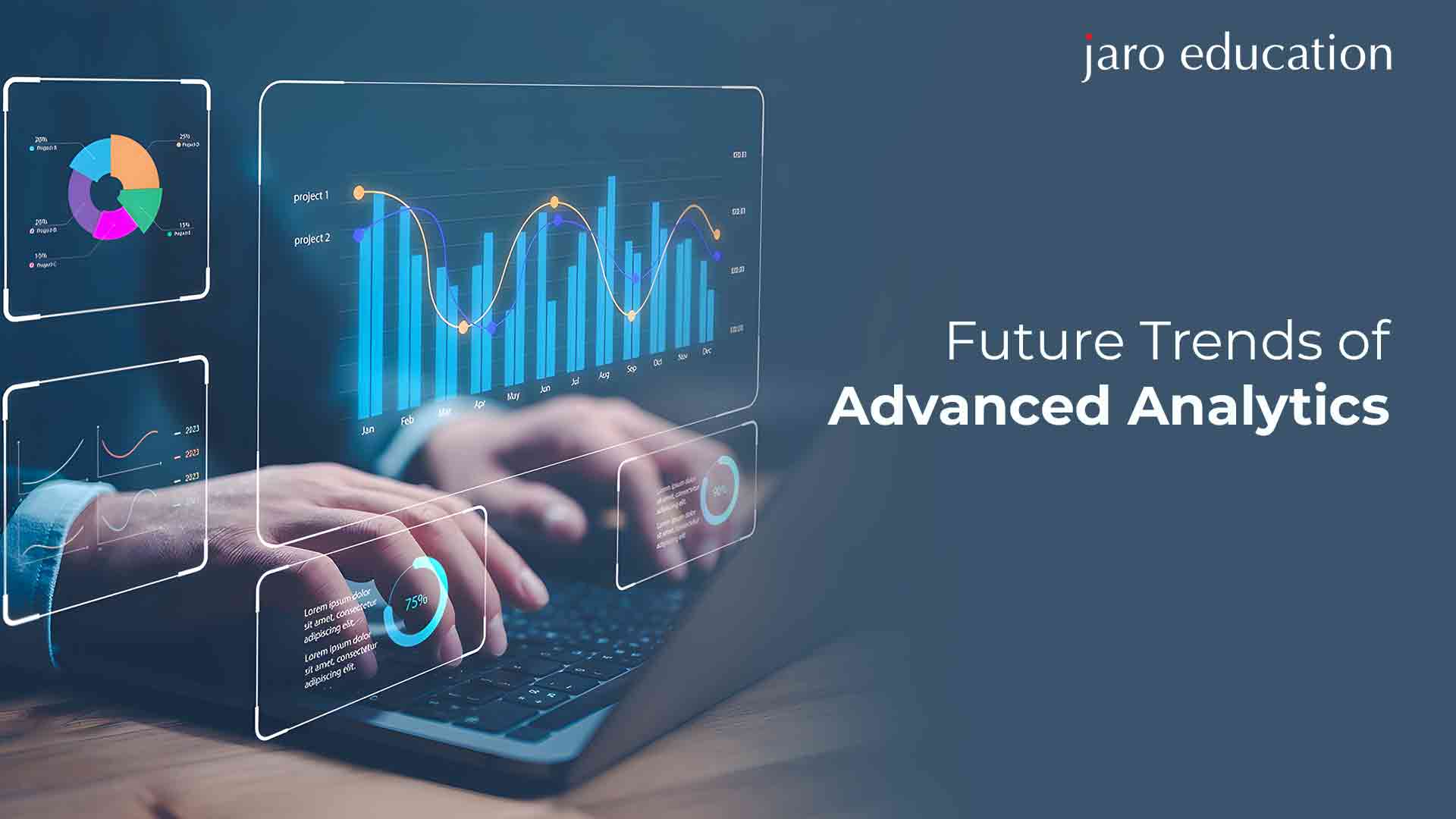
Future Trends in Machine Learning for Business Analytics
AI-Powered Business Intelligence Tools
As we look toward the future, one of the most exciting trends in machine learning is the development of AI-powered business intelligence (BI) tools. These advanced tools are changing the way organizations analyze data, offering unprecedented capabilities that drive decision-making. In essence, AI-powered BI tools can automate complex data analysis processes, making insights accessible to users across various levels of expertise. Imagine a marketing professional who traditionally relied on data analysts to interpret complex datasets. With AI-driven insights, they can now interact with the data directly, generating actionable findings almost instantly. Here are a few key advantages of AI-powered BI tools:
- Enhanced Data Visualization: Next-gen BI tools utilize machine learning to create dynamic and interactive dashboards. These visualizations can automate the storytelling aspect of data, allowing users to extract insights effortlessly.
- Predictive Analytics Integration: These tools can integrate predictive analytics capabilities that help businesses identify future trends based on historical data. For example, sales forecasts can be generated with a high degree of accuracy, enabling better resource allocation.
- Natural Language Processing (NLP): By incorporating NLP, these tools allow users to query data in conversational language. This means even those without technical expertise can ask complex data questions and receive straightforward answers, democratizing access to insights.
During my tenure at a startup, we adopted an AI-powered BI tool that transformed our reporting processes. Marketing teams could now generate detailed performance reports with minimal guidance, allowing us to make quick, informed decisions based on real-time data. This adaptability can significantly enhance the speed and accuracy of decision-making across an organization.
Adoption of Machine Learning in Small Businesses
Another notable trend shaping the future of machine learning in business analytics is its growing adoption among small businesses. Traditionally, small companies lacked the resources or expertise to implement complex machine learning solutions. However, advancements in technology have made these tools more accessible and cost-effective. Here’s how small businesses are leveraging machine learning to their advantage:
- Cost-Effective Solutions: With cloud-based machine learning services and open-source solutions, small businesses can access affordable tools that once required significant capital investment. This allows them to compete effectively against larger enterprises.
- Streamlined Operations: Machine learning algorithms can help small businesses automate routine tasks, from customer inquiries to inventory management. This not only reduces operational costs but also frees up time for employees to focus on strategic initiatives.
- Improved Customer Insights: By adopting machine learning, small businesses can gain valuable insights into customer behavior, helping them tailor products and services to meet specific needs. For instance, a local bakery could use machine learning to analyze customer purchase patterns and optimize its offerings based on popular items.
I recently spoke with the owner of a small e-commerce platform who implemented machine learning to improve customer segmentation. The insights gained helped him create targeted email campaigns that boosted conversions by over 25%. Such success stories are inspiring a wave of small businesses to embrace these innovative technologies. In conclusion, the future of machine learning in business analytics is bright, with AI-powered BI tools and increased adoption among small businesses leading the charge. As organizations continue to embrace these advancements, the focus on data-driven decision-making will become even more pronounced, reinforcing the importance of analytics in achieving sustainable growth and competitive advantage. Companies of all sizes must stay agile and open-minded to harness the potential of machine learning effectively, positioning themselves at the forefront of the evolving business landscape.